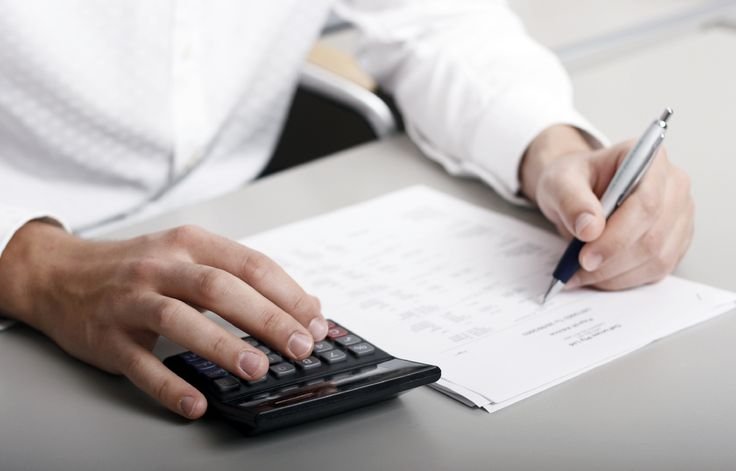
The financial insights space is getting disrupted. Once the traditional realm of debt levels, credit scores, and financial ratios – now moving to a data-centric approach. This evolution reflects wider changes in technology, analytics, and customer expectations. In this new analysis, we discuss recent research and trends helping push this movement toward the evolution from debt-centric models to a more complete data-driven paradigm.
The Old Way: A Debt-Centric Strategy
Debt and credit metrics have been the dominant narratives over financial insights for decades. Key indicators included:
Learn More Debt-to-Income Ratio: Measures the percentage of a person’s income for paying debts.
Credit Scores: These are numbers that represent the creditworthiness of an individual based on their credit history.
Leverage Ratios – metrics like debt to equity ratio, which shows how much of a company is financed by debt.
Those metrics are useful for measuring financial health, gauging risk, and ensuring your debt obligations are in check. But they present a somewhat narrow view, often looking only at your financial past and not into the future.
Evolution from Server-Centric to Data-Centric Insights
More recently, research has focused on using a wider range of data sources and better data to understand financial health and behavior. This move is due to new technological advances, more data at our fingertips, and changing consumer demands. Here is how that transformation is happening:
Big Data Analytics
The facility associated with the use of big data analytics has ensured that there is a vast overall knowledge of financial insights! Big data analyses enormous amounts of complex data, and it helps detect large amounts of hidden trends, patterns, or connections in traditional ways; a McKinsey & Company report by 2023 indicated that increased usage of big data analytics enables businesses to make better decisions produce more customer value and get the ultimate financial performance.
For instance, financial services providers are analyzing transaction data and spending patterns (and now even social media in some cases) to evaluate credit risk and behaviors. This enables more sophisticated risk evaluations and personalized financial services.
Alternative Data Sources
What is: Alternative data relates to non-traditional information that can be used to determine financial well-being and credit risk. However, it has become increasingly clear in recent studies, particularly from the World Bank, that there is real power behind alternative data sources. Suppose you have utility payment records consistent for years because hook-up costs make switching nearly prohibitively expensive. In that case, rental history is drawn from credit reports, locally relevant databases (e.g., public registries), or online behavior.
Cash Flow Data Inputs: Utility and Rental PaymentsUsers — Regular payments for utilities and rent can tell a great deal about someone’s reliability, stability, and financial health, which may be distinct from that captured in traditional credit data.
Social Media Metrics: The biggest role here is the social media metrics, which can be used to compare users to know how much they spend and their lifestyle, those influencing credit analysis or marketing.
Data in the Moment and Predictive Analysis
With real-time data, financial institutions can use predictive analytics that change decisions. Up-to-date information on financial behaviors and conditions provides immediate insights, which can be quickly counteracted to adapt to changing situations. Contrarily, Predictive analytics mines past data to predict what is likely to happen next.
In a study from 2024, Deloitte found that predictive analytics was very important in increasing risk management, with the ability to predict ahead of time about future financial troubles.cm For example, the banks can use predictive models to identify customers more likely to default on a loan to mitigate a worse scenario, like doing the action of their delinquent clients with them at risk of being defaulters.
Custom Financial Insights
This shift towards data-centric insights is also fuelling personalization in financial services. A comprehensive data-set analysis allows financial institutions to customize their advice, products, and services based on individual requirements and requests.
Financial products: Customized investment portfolios or tailor-made loans based on personal data and needsEHICLE PURCHASE RANGES BPerson PREFERENCES — HYBRIDCLUDING ELECTRICITY BEHIND HOME CH Structure
Specialized Financial Advice — financial advisors can come up with sharper advice and subsequent actions based on sophisticated data, delivering better outcomes to clients (and increasing client retention)
Advantages of the Data-Centric Approach
Enhanced Risk Assessment
A data centric approach gives us a finer granularity in assessing financial risks. Financial institutions can deepen their understanding of risk by integrating varying data points with the most up-to-date analytics. For instance, a 2023 report from the International Monetary Fund (IMF) demonstrated that risk models based on data could provide additional statistical power to forecasts of financial crises and defaults.
Improved Financial Inclusion
Alternative Data and Advanced Analytics: Beyond offering a gateway for otherwise difficult-to-verify applicants, these innovations could expand access to financial services by establishing creditworthiness among those without credit histories.
- Informed Decision-Making
The availability of comprehensive data allows for more informed decision-making. Financial institutions, investors, and consumers can make better decisions based on a holistic view of financial health and behavior. This leads to more effective financial planning, investment strategies, and risk management.
- Increased Efficiency
Automated data analysis and real-time insights enhance operational efficiency. Financial institutions can streamline processes, reduce manual interventions, and improve financial reporting and decision-making accuracy.
Challenges and Considerations
- Data Privacy and Security
The reliance on extensive data raises concerns about privacy and security. Ensuring that personal and financial data is protected from breaches and misuse. Research by the Data Protection Commissioner emphasizes the need for robust data protection measures and compliance with privacy regulations.
- Data Quality and Integration
Integrating and analyzing diverse data sources can be challenging. Ensuring data quality and consistency is essential for accurate insights. A 2024 study from the European Central Bank highlights the importance of data governance and quality control in achieving reliable and actionable financial insights.
- Regulatory and Ethical Concerns
The use of alternative data and advanced analytics raises regulatory and ethical questions. Regulators scrutinize how data is used and how decisions are made based on these insights. The 2023 guidelines from the Financial Stability Board emphasize the need for transparent and fair practices in data-driven financial analysis.
Future Directions
- Advancements in Artificial Intelligence (AI)
Integrating AI with data analytics is expected to drive further advancements in financial insights. AI algorithms can enhance predictive analytics, automate decision-making processes, and uncover complex patterns in financial data. Research from MIT Sloan School of Management highlights the transformative potential of AI in financial services.
- Evolving Data Regulations
As data usage evolves, regulatory frameworks will need to adapt. Future regulations will likely focus on balancing innovation with privacy and security concerns. Ongoing research into data governance and regulation will shape how financial institutions navigate these challenges.
- Integration of Emerging Technologies
The convergence of emerging technologies, such as blockchain and the Internet of Things (IoT), with data analytics will create new opportunities for financial insights. Blockchain technology can enhance data security and transparency, while IoT devices can provide real-time data on financial transactions and behaviors.
Leave a Reply